Building upon our previous discussion in Part 1, where we unpacked the complexities and limitations of Predictive Coding in eDiscovery, Part 2 of our series shifts focus to Continuous Active Learning (CAL). This segment intends to explore how CAL, as an advanced iteration of Technology-Assisted Review (TAR), addresses some of the challenges identified earlier while introducing its own unique set of considerations. We will navigate through the intricacies of CAL, examining its impact on the efficiency and accuracy of the eDiscovery process and how it reshapes our approach to legal technology.
Continuous Active Learning
Traditional Technology-Assisted Review (TAR) methods, such as Simple Active Learning (SAL) or Simple Passive Learning (SPL), have given way to Continuous Active Learning (CAL) due to their adaptability and efficiency in many eDiscovery contexts. CAL involves continuous training of the machine learning model throughout the review process. However, despite its advantages, CAL presents several potential pitfalls and challenges, especially in document review in eDiscovery.
Drift in Document Population
Drift in Document Population:
As the Review progresses, the nature of the remaining unreviewed documents can change, potentially making the latter stages of the Review more challenging. This drift can impact the efficiency of the model. The model may become less accurate if not correctly updated to account for the drift. The drift may cause the model to miss crucial documents that become relevant later in the review process.
Reviewer Inconsistency
In a continuous active learning environment, the model relies heavily on the consistency of human reviewers. If different reviewers have varying interpretations of relevance or a single reviewer’s criteria change over time, it can confuse the machine learning model. The model might receive mixed signals, leading to reduced accuracy. Inconsistent Coding can lead to inefficiencies and increased costs due to re-reviews.
Risk of Overfitting
In CAL, the model is constantly being trained on newly labeled data. Suppose the review team focuses too narrowly on a particular document or issue. In that case, the model can become over-specialized or overfit to that data, thereby missing other relevant documents. It will reduce the model’s generalizability and potentially overlook significant sets of documents not represented in the continuous training data.
Quality Control Challenges
CAL’s dynamic nature can make traditional quality control measures less effective. Since the model constantly evolves, ensuring consistent quality throughout the Review is challenging and will require more frequent and adaptable quality checks. It will also increase the complexity of the review process.
Effort and Time Intensity
The system might frequently present borderline documents to reviewers for Coding in CAL. This can be mentally taxing, requiring more effort and time than reviewing relevant or irrelevant documents. Increases reviewer fatigue, potentially reducing accuracy over time. It may slow down the review process due to challenging decisions.
Dependence on Initial Seed Set
Though CAL diminishes the reliance on the initial seed set compared to other TAR methods, the starting set of documents still plays a role. This seed set can influence the model’s direction if it is not carefully chosen. It can create potential biases in the initial stages of model training. The necessity for a well-curated seed set adds to the process’s complexity.
Scalability Concerns
As data grows, continuously training a model can become computationally intensive. CAL requires robust infrastructure, especially when dealing with vast datasets. This may lead to increased costs due to computational requirements. Requires continuous monitoring to ensure the system runs smoothly.
Continuous Active Learning offers a more adaptive and efficient approach to eDiscovery document review compared to older TAR methods. However, it introduces challenges that legal and technological professionals must be aware of and address. Proper training, infrastructure, and quality control measures are essential to harness CAL’s strengths while navigating its pitfalls.
In wrapping up our exploration of Continuous Active Learning, we’ve unraveled the complexities accompanying this advanced eDiscovery approach. The challenges, from managing evolving document populations to ensuring scalable and efficient model training, underscore the need for meticulous planning and execution. As we transition to the final part of our series, we will shift our focus to the future of eDiscovery: the promising role of Large Language Models (LLMs) in revolutionizing document review processes, offering a glimpse into a more autonomous and sophisticated legal technology landscape.
About the Author
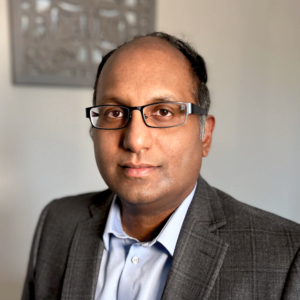
VASUDEVA MAHAVISHNU
Vasudeva is the CTO at Altumatim. Vasu brings his natural curiosity and passion for using technology to improve access to justice and our quality of life to the Altumatim team as he architects and builds out the future of discovery. Vasu blends computer science and data science expertise from computational genomics with published work ranging from gene mapping to developing probabilistic models for protein interactions in humans.